Analog sensors are all over the place and can be used to gauge temperatures, pressure as well as power levels and many more. Because of the introduction electronic sensors a lot were quickly outdated and left the rest to be converted to digital format or constant human monitoring. However Zalmotek’s Zalmotek team has developed an idea that combines embedded computer vision and machine learning to read autonomously these values.
In an enclosure that they designed The project uses the Arduino Pro Nicla Vision board that captures periodic images to further process and inference. They started by creating the fake gauge images that show the dial in different places, and then they labeled them as normal, low or high. The collection was then loaded in Edge Impulse Studio. Edge Impulse Studio and used to build a machine learning model on the 96x96px images due to the memory limitations. Once the neural network was able to detect the gauge’s condition about 92 percent of the time.
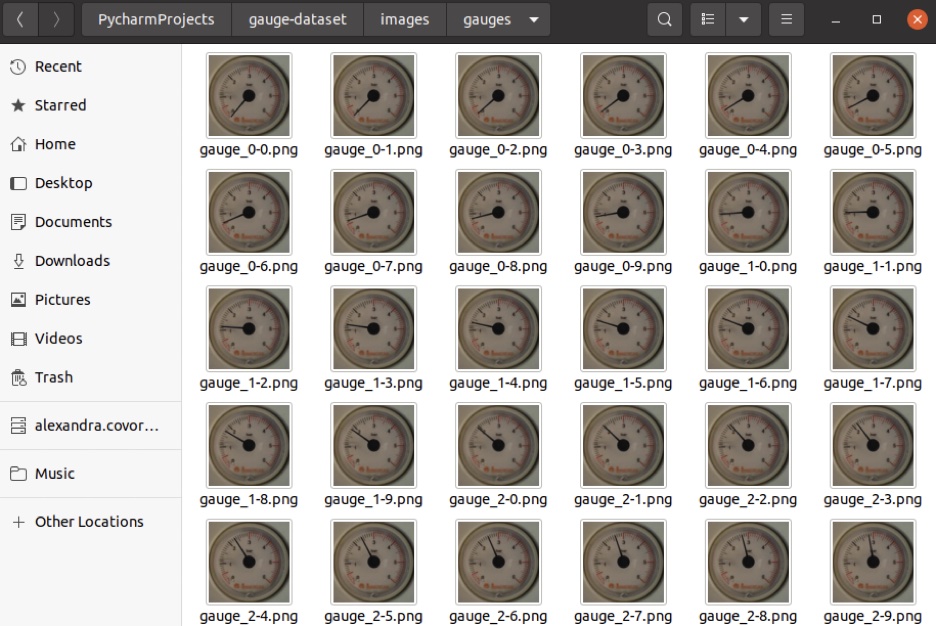
The final step of this project involved deploying the firmware to the Nicla Vision and setting the image size to the aforementioned 96x96px size. By opting to use this technique of computer vision, frequent readings can be taken while also minimizing cost and power consumption.
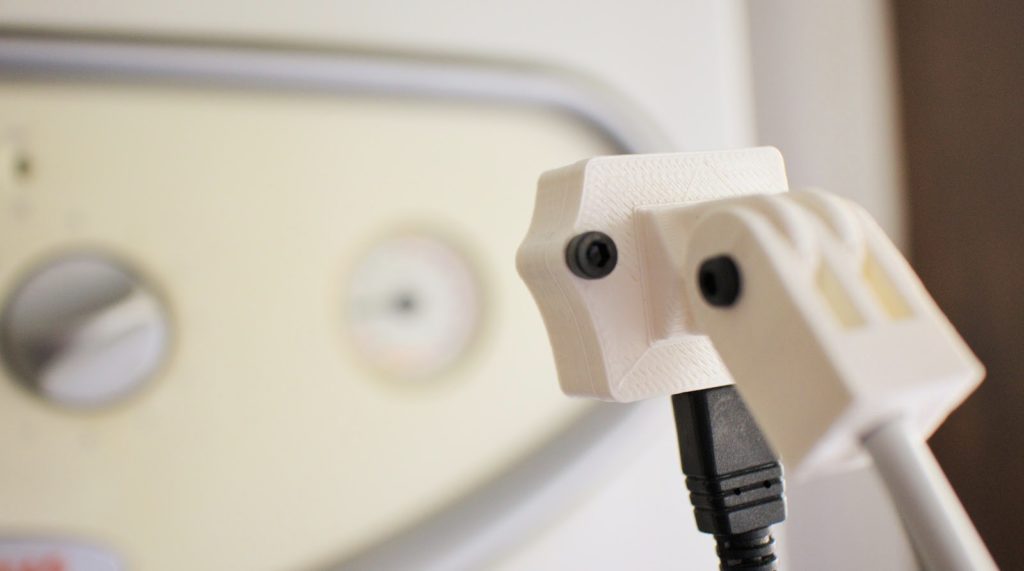
More details on Zalmotek’s system can be found here on its Edge Impulse docs page.